
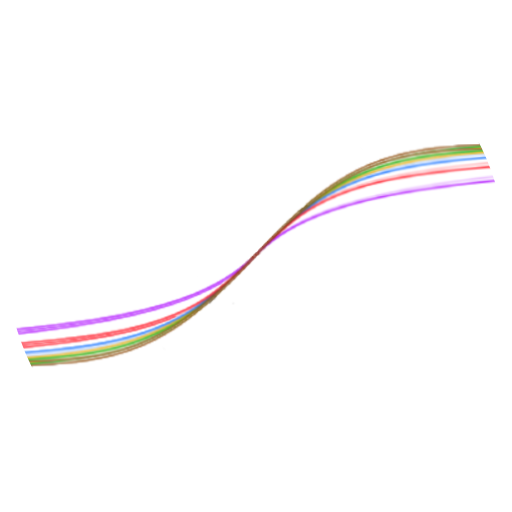
I expected that recording would be the hard part.
I think some of the open-source ones should work if your phone is rooted?
I’ve heard that Google’s phone app can record calls (though it says it aloud when starting the recording). Of course, it wouldn’t work if Google thinks it shouldn’t in your region.
By the way, Bluetooth headphones can have both speakers and a microphone. And Android can’t tell a peripheral device what it should or shouldn’t do with audio streams. Sounds like a fun DIY project if you’re into it, or maybe somebody sells these already.
LLaMA can’t. Chameleon and similar ones can: